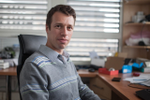
HANGYA Balázs
- Lendület Laboratory of Systems Neuroscience, Institute of Experimental Medicine, Budapest, Hungary
- Electrophysiology, Interneurons, Systems/Circuit Neuroscience, Neuronal oscillations
- recommender
Recommendations: 2
Review: 1
Recommendations: 2
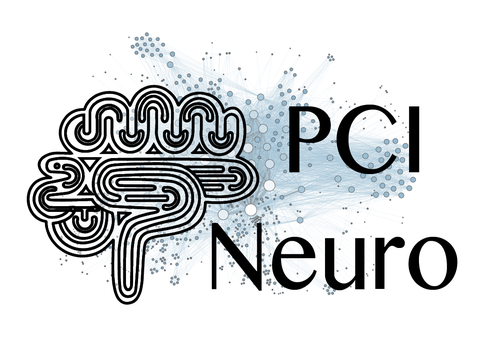
Multisensory coding of angular head velocity in the retrosplenial cortex
Where was I going? Vestibular-visual integration in the retrosplenial cortex
Recommended by Balázs Hangya and Koen VervaekeWe need to keep track of our heading direction, and head direction cells in various brain regions represent exactly that; therefore, they have been identified as key substrates of direction coding. But how is such a variable computed? Head direction representation is thought to arise from the integration of angular velocity, suggesting the existence of angular head velocity (AHV) neurons. Theoretically, vestibular, visual, proprioceptive and motor command signals can all contribute to angular velocity computations, while the actual coding mechanisms have remained unclear.Keshavarzi et al. addressed this by separately controlling vestibular and visual cues in a head-fixed configuration (Keshavarzi et al., 2022b). They targeted the retrosplenial cortex (RSC), a multisensory associational area known to be important for the integration of egocentric and allocentric information during navigation (Solari & Hangya, 2018). The use of high channel-count multielectrode arrays (silicon probes) allowed the authors to track a large number of neurons through different conditions, including free exploration of an open field arena. They identified subsets of neurons representing head direction, AHV, locomotion speed or a combination of these variables.
A large fraction of AHV neurons showed similar tuning properties during free exploration and passive rotation in the dark, demonstrating the importance of vestibular input. In agreement, lesioning the semi-circular canals largely reduced these responses. Next, mice were presented with visual motion stimuli while remaining stationary. This showed that visual signals also contributed to AHV coding and specifically suggested that they increased the gain and improved the signal-to-noise ratio of AHV representations. Mice trained on discriminating rotational stimuli showed improved performance when both visual and vestibular information was available compared to either vestibular-only or visual-only stimuli, further demonstrating the integration of the two types of sensory cues. Finally, the availability of both vestibular and visual information improved decoding accuracy of angular speed from ensembles of retrosplenial cortex neurons compared to single modality stimuli, at least at the beginning of motion.
Keshavarzi et al. provide compelling evidence for the critical role of vestibular input in encoding AHV within the RSC. While the widespread presence of vestibular signals in rodent cortical circuits is well-documented (Rancz et al., 2015), this study significantly advances our understanding by demonstrating that RSC neurons can also encode AHV. These findings align with research that identified AHV representations in the RSC and adjacent cortical regions, including primary visual, secondary visual, posterior parietal, primary motor, secondary motor and primary somatosensory cortices (Hennestad et al., 2021), and with later work that showed AHV in parahippocampal circuits (Spalla et al., 2022).
Notably, the relative contributions of vestibular and visual signals to AHV encoding likely depend on the specific cortical area and the AHV amplitude. Visual flow may be more prominent at lower AHV ranges (0-90 deg/s), while vestibular input likely dominates AHV representation at higher speeds (Stahl, 2004; Hennestad et al., 2021). This suggests a complementary contribution of vestibular and visual information, enabling encoding a broader range of angular velocity and driving a widespread AHV signal across cortical areas.
This is an elegant study in which a creative and clear experimental design helps teasing apart different contributors of a specific computation that normally appear linked during natural behaviors. This way it also demonstrates the power of precise experimental control, while immediately extrapolating to natural behavior by examining the same neurons during free exploration. It additionally demonstrates a non-trivial multisensory integration in the retrosplenial cortex that can directly contribute to egocentric spatial representations during navigation (Alexander & Nitz, 2015, 2017).
Editorial note: A preprint version of this article was peer-reviewed by PCI Neuroscience. The refereed preprint can be found through the cited link here (Keshavarzi et al., 2022a), and the peer-review process here.
References
Alexander AS, Nitz DA (2015) Retrosplenial cortex maps the conjunction of internal and external spaces. Nature Neuroscience, 18, 1143–1151. https://doi.org/10.1038/nn.4058
Alexander AS, Nitz DA (2017) Spatially Periodic Activation Patterns of Retrosplenial Cortex Encode Route Sub-spaces and Distance Traveled. Current Biology, 27, 1551-1560.e4. https://doi.org/10.1016/j.cub.2017.04.036
Hennestad E, Witoelar A, Chambers AR, Vervaeke K (2021) Mapping vestibular and visual contributions to angular head velocity tuning in the cortex. Cell Reports, 37, 110134. https://doi.org/10.1016/j.celrep.2021.110134
Keshavarzi S, Bracey EF, Faville RA, Campagner D, Tyson AL, Lenzi SC, Branco T, Margrie TW (2022a) The retrosplenial cortex combines internal and external cues to encode head velocity during navigation. https://doi.org/10.5281/zenodo.5834392
Keshavarzi S, Bracey EF, Faville RA, Campagner D, Tyson AL, Lenzi SC, Branco T, Margrie TW (2022b) Multisensory coding of angular head velocity in the retrosplenial cortex. Neuron, 110, 532-543.e9. https://doi.org/10.1016/j.neuron.2021.10.031
Rancz EA, Moya J, Drawitsch F, Brichta AM, Canals S, Margrie TW (2015) Widespread vestibular activation of the rodent cortex. The Journal of Neuroscience: The Official Journal of the Society for Neuroscience, 35, 5926–5934. https://doi.org/10.1523/JNEUROSCI.1869-14.2015
Solari N, Hangya B (2018) Cholinergic modulation of spatial learning, memory and navigation. European Journal of Neuroscience, 48, 2199–2230. https://doi.org/10.1111/ejn.14089
Spalla D, Treves A, Boccara CN (2022) Angular and linear speed cells in the parahippocampal circuits. Nature Communications, 13, 1907. https://doi.org/10.1038/s41467-022-29583-z
Stahl JS (2004) Using eye movements to assess brain function in mice. Vision Research, 44, 3401–3410. https://doi.org/10.1016/j.visres.2004.09.011
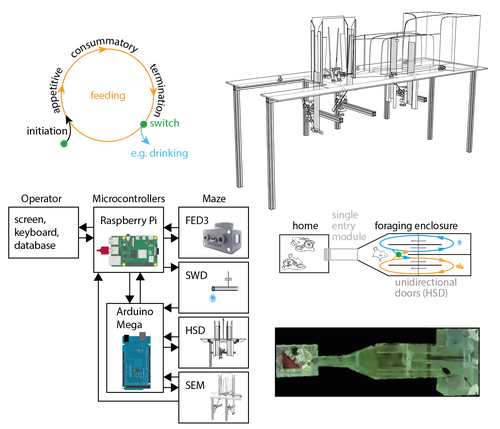
The Switchmaze: an open-design device for measuring motivation and drive switching in mice
Novel automated training platform for studying flexible switching among natural motivated behaviors in mice
Recommended by Balázs Hangya based on reviews by Ede Rancz and Ewelina KnapskaAs our understanding of the building blocks of mammalian behavior improves, there is a shifting focus towards addressing the brain mechanisms of behavioral flexibility, strategy learning and behavioral switching (Banerjee et al., 2020; López-Yépez et al., 2021; Manzur et al., 2023). This requires novel behavioral paradigms and new tools: Hartmann and colleagues started filling this gap by presenting an open-source automated training system for studying motivational switching, which they coined the ‘Switchmaze’ (Hartmann et al., 2024).
Instead of training mice on specific tasks, Hartmann et al. chose to quantify switching between spontaneous motivated behaviors like feeding, drinking, and engaging in social interactions in a type of foraging task. These behaviors were spatially separated by a smart design using distinct compartments with unidirectional doors, allowing the counting of discrete cycles of food and water intake in unitary quantities.
Switching behavior was quantified by the ratio of shifting from one behavioral chamber to another (single probe entries) versus exploitation of a single chamber through multiple consecutive entries (continuous exploitation runs), termed ‘motivation switching rate’ (MSR). Interestingly, the measured MSR values were well within the distribution of randomized data in which the trial sequence was shuffled. The Authors suggest that this may be an adaptive strategy to decrease behavioral predictability and thus fool competitors and predators; however, determining the significance of this finding will require further testing. For instance, is a ‘more random mouse’ indeed more successful in a competitive setting where the total amounts of food and water are limited? Food deprivation increased, while re-feeding decreased switch rate, strengthening the arguments for the MSR being a strategically controlled parameter.
Hartmann et al. further demonstrated the utility of the Switchmaze by performing chemogenetic inhibition of prefrontal cortical neurons projecting to the hypothalamus, a pathway thought to be involved in controlling feeding behavior (Petrovich et al., 2005; Cole et al., 2020; Padilla-Coreano et al., 2022). Mice showed an increased MSR upon inhibition, now significantly different from randomized distributions. Further analysis revealed that the difference was driven by a selective reduction of food-to-food transitions, that is, a decreased tendency for repetitive feeding. Moreover, this was due to a decrease in the number but not the duration of food runs, suggesting a specific behavioral role of the prefrontal-hypothalamic pathway in promoting repetitive feeding.
In summary, Hartmann and colleagues showcased an affordable, open-source behavioral design and demonstrated its usefulness for quantifying flexible switching of natural behaviors. It is ideal for testing the effect of pharmacological and chemogenetic manipulations, but it can likely be combined with electrophysiology, fiber photometry or miniscope imaging, greatly broadening its potential. Therefore, the Switchmaze is a valuable member of the growing family of open source, automated rodent training tools (Puścian et al., 2016; Erskine et al., 2019; Qiao et al., 2019; Birtalan et al., 2020; Cano-Ferrer et al., 2024) that represent the logical next step for high-throughput, stress- and bias-free behavioral experimentation.
References
Banerjee A, Parente G, Teutsch J, Lewis C, Voigt FF, Helmchen F (2020) Value-guided remapping of sensory cortex by lateral orbitofrontal cortex. Nature 585:245–250 Available at: http://dx.doi.org/10.1038/s41586-020-2704-z.
Birtalan E, Bánhidi A, Sanders JI, Balázsfi D, Hangya B (2020) Efficient training of mice on the 5-choice serial reaction time task in an automated rodent training system. Sci Rep 10:22362 Available at: https://doi.org/10.1038/s41598-020-79290-2.
Cano-Ferrer X, Tran-Van-Minh A, Rancz E (2024) RPM: An open-source Rotation Platform for open- and closed-loop vestibular stimulation in head-fixed Mice. J Neurosci Methods 401:110002 Available at: https://doi.org/10.1016/j.jneumeth.2023.110002.
Cole S, Keefer SE, Anderson LC, Petrovich GD (2020) Medial Prefrontal Cortex Neural Plasticity, Orexin Receptor 1 Signaling, and Connectivity with the Lateral Hypothalamus Are Necessary in Cue-Potentiated Feeding. J Neurosci 40:1744–1755 Available at: https://www.jneurosci.org/lookup/doi/10.1523/JNEUROSCI.1803-19.2020.
Erskine A, Bus T, Herb JT, Schaefer AT (2019) AutonoMouse: High throughput operant conditioning reveals progressive impairment with graded olfactory bulb lesions Reisert J, ed. PLoS One 14:e0211571 Available at: https://dx.plos.org/10.1371/journal.pone.0211571.
Hartmann C, Mahajan A, Borges V, Razenberg L, Thönnes Y, Karnani MM (2024) The Switchmaze: an open-design device for measuring motivation and drive switching in mice. bioRxiv:1–17 Available at: https://doi.org/10.1101/2024.01.31.578188.
López-Yépez JS, Martin J, Hulme O, Kvitsiani D (2021) Choice history effects in mice and humans improve reward harvesting efficiency Palminteri S, ed. PLOS Comput Biol 17:e1009452 Available at: https://dx.plos.org/10.1371/journal.pcbi.1009452.
Manzur HE, Vlasov K, Jhong Y-J, Chen H-Y, Lin S-C (2023) The behavioral signature of stepwise learning strategy in male rats and its neural correlate in the basal forebrain. Nat Commun 14:4415 Available at: https://www.nature.com/articles/s41467-023-40145-9.
Padilla-Coreano N et al. (2022) Cortical ensembles orchestrate social competition through hypothalamic outputs. Nature 603:667–671 Available at: https://www.nature.com/articles/s41586-022-04507-5.
Petrovich GD, Holland PC, Gallagher M (2005) Amygdalar and Prefrontal Pathways to the Lateral Hypothalamus Are Activated by a Learned Cue That Stimulates Eating. J Neurosci 25:8295–8302 Available at: https://www.jneurosci.org/lookup/doi/10.1523/JNEUROSCI.2480-05.2005.
Puścian A, Łęski S, Kasprowicz G, Winiarski M, Borowska J, Nikolaev T, Boguszewski PM, Lipp H-P, Knapska E (2016) Eco-HAB as a fully automated and ecologically relevant assessment of social impairments in mouse models of autism. Elife 5:1–22 Available at: https://elifesciences.org/articles/19532.
Qiao M, Zhang T, Segalin C, Sam S, Perona P, Meister M (2019) Mouse Academy: high-throughput automated training and trial-by-trial behavioral analysis during learning. bioRxiv:467878 Available at: http://biorxiv.org/content/early/2019/02/13/467878.abstract.
Review: 1
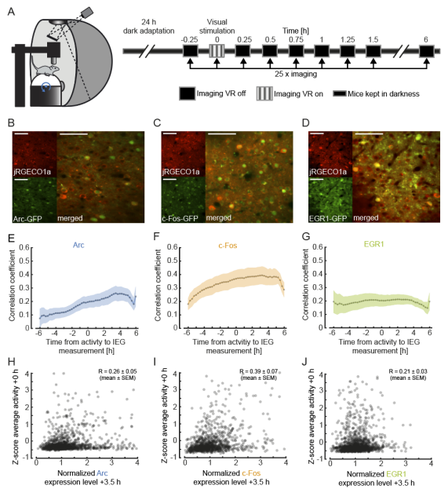
Functional correlates of immediate early gene expression in mouse visual cortex
Bringing together immediate early genes and sensorimotor response properties in V1
Recommended by Julia Jade Harris and Sepiedeh Keshavarzi based on reviews by Balázs Hangya and 2 anonymous reviewersThe primary visual cortex (V1) does not just process vision: it also integrates self-generated motion signals (Niell, Stryker 2010; Keller et al. 2012; Saleem et al. 2013; Vélez-Fort et al. 2018; Meyer et al. 2018), enabling us to match our actions to the world we see. We know that the development of visuomotor representation in V1 depends on experience (Attinger et al. 2017; Widmer et al. 2022), but how exactly does each neuron acquire the right balance of visual and motor input? And how do some neurons become more responsive to visual or motor signals? Mahringer et al. (Mahringer et al. 2022) suspected that the answers may lie in experience-specific plasticity mechanisms.
To investigate this, the authors measured the expression of immediate early genes (IEGs) as indicators of both past neural activity and future plasticity. They examined three IEGs previously implicated in visual cortical plasticity: c-fos, egr1 and Arc (Yamada et al. 1999; Wang et al. 2006; Xie et al. 2014). In three separate transgenic mouse lines, GFP expression was driven by these IEGs, and a red variant of a genetically encoded calcium indicator allowed for simultaneous measurement of neuronal activity. Initial characterisation of IEG expression and calcium fluorescence revealed that IEG levels were only weakly (positively) correlated with visually-evoked neural activity.
But what about the relationship between IEG expression and first visual or visuomotor experience? In dark-reared mice, first visual and visuomotor experiences led to differential IEG expression: Arc expression increased after first visual and visuomotor experiences; EGR1 expression decreased after first visuomotor experience; and c-Fos expression remained largely unchanged. Neural activity levels could not account for these changes, suggesting that different sensory experiences can selectively recruit different IEG expression patterns, perhaps according to input pathway.
Further analysis of those neurons with the highest levels of IEG expression revealed that different IEGs were associated with different functional response properties. High Arc-expressing neurons developed above-average visual and below-average motor responses, while high EGR1-expressing neurons developed above-average motor responses. These results suggest that during experience-dependent wiring, Arc expression drives plasticity favouring bottom-up visual input, while EGR1 expression drives plasticity favouring top-down motor input. Interestingly, while Arc-expressing neurons appear to end up with little-to-no motor input, EGR1-expressing neurons appear to enjoy both visual and motor input, enabling them to display above-average visuomotor mismatch responses.
Overall, this work makes two important advances. First, it suggests that IEG expression may be more closely linked to specific forms of plasticity than general levels of neural activity. Second, it reveals a mechanism by which visual cortical neurons can acquire specific functional properties by selectively upregulating bottom-up or top-down inputs in response to particular sensory experiences.
As an additional note, we would like to highlight a vigorous technical discussion that this manuscript triggered: unconventionally, the authors chose not to apply a neuropil correction procedure to their calcium imaging data. This decision split opinion, amongst both reviewers and recommenders. We have come to the view that the findings are nevertheless of interest for the community and are pleased to point readers towards the publicly available reviews and authors’ responses.
Attinger A, Wang B, Keller GB (2017) Visuomotor Coupling Shapes the Functional Development of Mouse Visual Cortex. Cell, 169, 1291-1302.e14. https://doi.org/10.1016/j.cell.2017.05.023
Keller GB, Bonhoeffer T, Hübener M (2012) Sensorimotor mismatch signals in primary visual cortex of the behaving mouse. Neuron, 74, 809–815. https://doi.org/10.1016/j.neuron.2012.03.040
Mahringer D, Zmarz P, Okuno H, Bito H, Keller GB (2022) Functional correlates of immediate early gene expression in mouse visual cortex. bioRxiv, 2020.11.12.379909, ver. 4 peer-reviewed and recommended by Peer community in Neuroscience. https://doi.org/10.1101/2020.11.12.379909
Meyer AF, Poort J, O’Keefe J, Sahani M, Linden JF (2018) A Head-Mounted Camera System Integrates Detailed Behavioral Monitoring with Multichannel Electrophysiology in Freely Moving Mice. Neuron, 100, 46-60.e7. https://doi.org/10.1016/j.neuron.2018.09.020
Niell CM, Stryker MP (2010) Modulation of Visual Responses by Behavioral State in Mouse Visual Cortex. Neuron, 65, 472–479. https://doi.org/10.1016/j.neuron.2010.01.033
Saleem AB, Ayaz A, Jeffery KJ, Harris KD, Carandini M (2013) Integration of visual motion and locomotion in mouse visual cortex. Nature Neuroscience, 16, 1864–1869. https://doi.org/10.1038/nn.3567
Vélez-Fort M, Bracey EF, Keshavarzi S, Rousseau CV, Cossell L, Lenzi SC, Strom M, Margrie TW (2018) A Circuit for Integration of Head- and Visual-Motion Signals in Layer 6 of Mouse Primary Visual Cortex. Neuron, 98, 179-191.e6. https://doi.org/10.1016/j.neuron.2018.02.023
Wang KH, Majewska A, Schummers J, Farley B, Hu C, Sur M, Tonegawa S (2006) In Vivo Two-Photon Imaging Reveals a Role of Arc in Enhancing Orientation Specificity in Visual Cortex. Cell, 126, 389–402. https://doi.org/10.1016/j.cell.2006.06.038
Widmer FC, O’Toole SM, Keller GB (2022) NMDA receptors in visual cortex are necessary for normal visuomotor integration and skill learning. eLife, 11, e71476. https://doi.org/10.7554/eLife.71476
Xie H, Liu Y, Zhu Y, Ding X, Yang Y, Guan J-S (2014) In vivo imaging of immediate early gene expression reveals layer-specific memory traces in the mammalian brain. Proceedings of the National Academy of Sciences, 111, 2788–2793. https://doi.org/10.1073/pnas.1316808111
Yamada Y, Hada Y, Imamura K, Mataga N, Watanabe Y, Yamamoto M (1999) Differential expression of immediate-early genes, c-fos and zif268, in the visual cortex of young rats: effects of a noradrenergic neurotoxin on their expression. Neuroscience, 92, 473–484. https://doi.org/10.1016/S0306-4522(99)00003-2